Introduction:
Images are essential to our life because they allow us to communicate, record events, and express ourselves creatively. The volume of visual data produced each day has skyrocketed due to the widespread use of digital cameras, cell phones, and social media platforms. We use image analysis to make sense of this massive amount of data. Computer science's field of image analysis tries to draw important conclusions from digital images so that we can comprehend, interpret, and work with visual data.
It uses various techniques and algorithms to analyze and interpret visual data, allowing for automated image processing, comprehension, and manipulation. Applications for image analysis can be found in many different industries, including robotics, surveillance, agriculture, Pharmaceutical and more. Many software companies like ImageProVision Technology are focusing on offering consumers tailor-made solutions and services to enhance image analysis services.
Image analysis is fundamentally the processing and interpretation of images through the use of algorithms and procedures. It includes a broad range of operations, such as picture augmentation, segmentation, feature extraction, object recognition, and image classification.
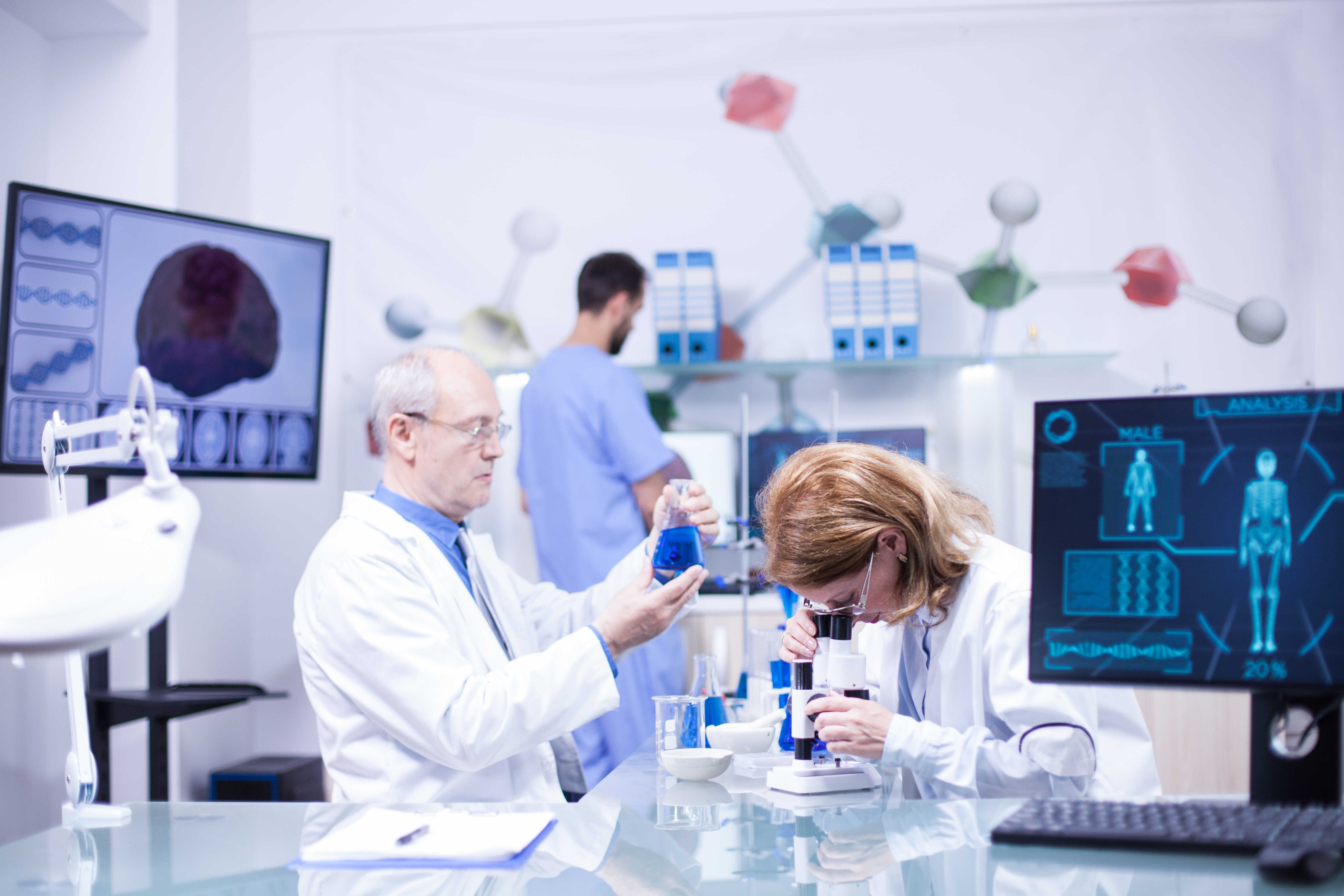
Following are some of the basic ideas in image analysis:
- Image Acquisition:
Before beginning image analysis, images must first be obtained from a variety of sources, including digital cameras, satellites, MRI machines, scanner and video feeds. The attributes and quality of the obtained photos can have a big impact on the rest of the study.
- Pre-processing:
Noise, artefacts, and consistency issues frequently plague raw photos. Images are pre-processed using methods like noise reduction, picture registration, and colour correction to enhance their quality and prepare them for future study.
- Segmentation:
Segmentation is the process of separating an image into useful areas or objects. By isolating particular areas of interest, making subsequent analysis tasks easier, and enabling object-level knowledge, it plays a crucial part in image analysis.
- Feature extraction:
Feature extraction is the process of identifying measurable aspects of an image that can be used to distinguish between various objects or regions. Different methods are used to extract features from photographs, such as texture, shape, colour, or spatial relationships.
- Object recognition:
Object recognition seeks to locate and categorise certain objects or patterns in a picture. Matching extracted features against a set of predetermined templates is required for this activity, or machine learning algorithms can be used for object detection and recognition.
- Image classification:
This entails categorising photographs into predetermined groups depending on the content of the images. By reaching astounding accuracy in tasks like facial recognition, object detection, and scene interpretation, machine learning techniques such as deep learning and convolutional neural networks (CNNs) have revolutionised the classification of images.
Read Also - The Basic Of Image Processing
Image Analysis Applications:
Applications for image analysis may be found in many industries, and they have a big impact on industries including healthcare, robotics, surveillance, agriculture, and entertainment.
Here are a few instances:
- Security and surveillance:
Image analysis is often used in surveillance systems for facial identification, object tracking, and video analytics. It assists in spotting suspicious activity, following things or people, and giving out instant alerts or notifications. Access control systems, crowd surveillance, transportation security, and public safety all benefit from the use of this technology.
- Robotics and automation:
Image analysis is crucial for operations like object detection, localization, and navigation in robotics and automation. Robots using cameras at their disposal can scan their environment, recognize objects, and make judgements based on visual information. Applications made possible by this include robotic help in numerous industries, driverless vehicles, industrial automation, and item sorting.
- Agriculture:
Image analysis can support precision agriculture through crop health monitoring, vegetation growth assessment, disease detection, and yield prediction. Farmers can make well-informed decisions about irrigation, fertilization, and pest control by examining aerial or satellite photos. This enhances agricultural productivity while maximizing resource use and minimizing negative environmental effects.
- Pharmaceutical Industry:
Microscopic image analysis system with a high-resolution camera and dedicated software integrated with the microscope is used for the identification of particulate solids, shapes, and textures within the microstructure of the sample. ipvPClass by ImageProVision is one such example of a microscopic image analysis system used in the pharmaceutical industry.
- Quality control and inspection:
Image analysis is used in the manufacturing sector to inspect and control the quality of items. It makes it possible to measure dimensions, check for product integrity, and discover flaws automatically. This maintains constant quality, lowers human error, and boosts the effectiveness of production.
- Biometrics:
To authenticate and verify identities, biometric systems use image analysis. Examples of biometric applications that depend on image analysis algorithms include palmprint identification, fingerprint scanning, iris recognition, and facial recognition. Security systems, access control, and personal gadgets like smartphones all use these technologies.
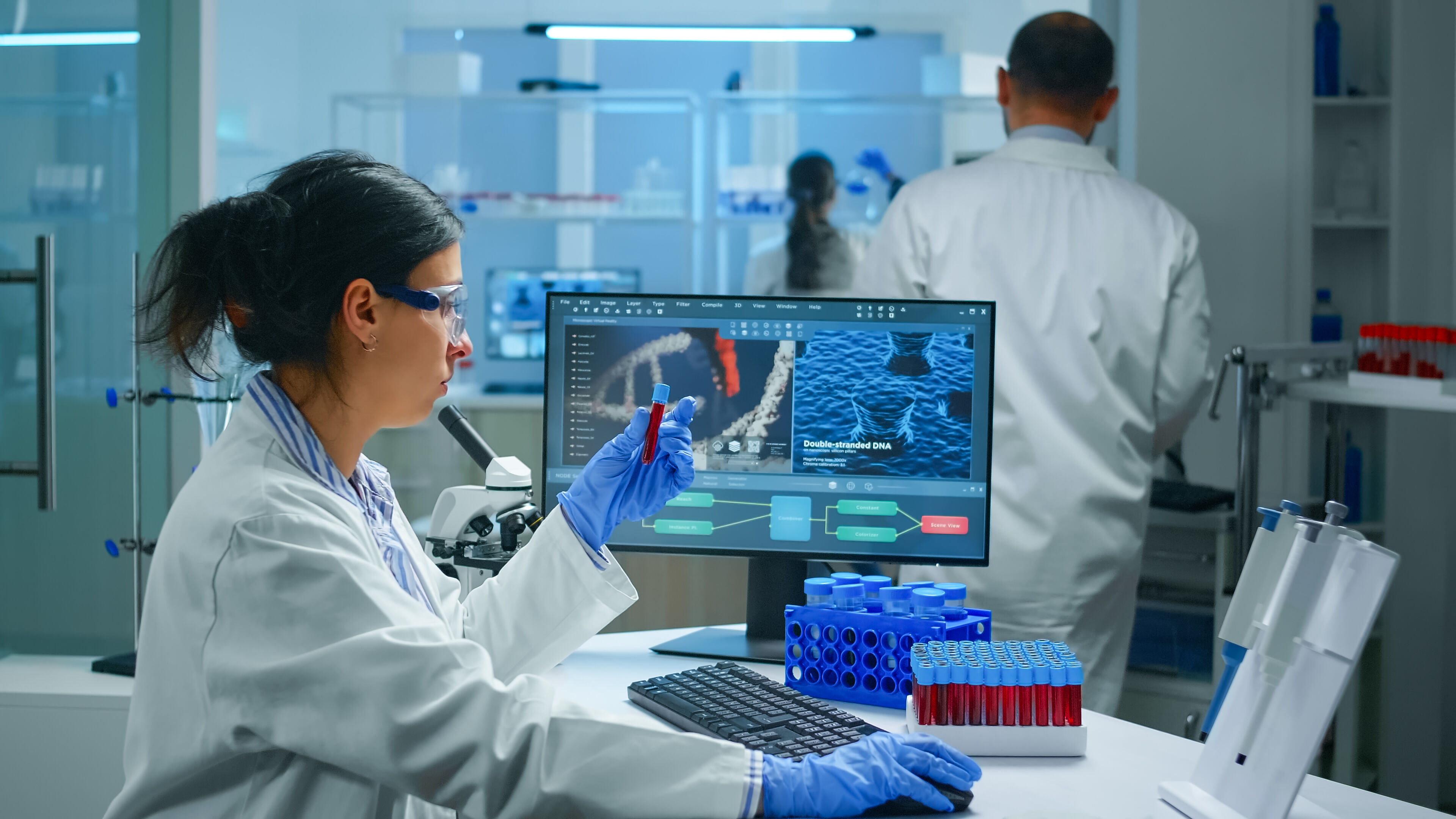
How Has Image Analysis Reshaped the Pharmaceutical Industry?
The pharmaceutical sector has seen a radical transformation thanks to image analysis, which has transformed several areas including research, development, production, quality assurance, and patient care.
Here are some examples of how image analysis has changed the pharmaceutical sector:
1. The drug development process has been sped up by image analysis tools that enable automated examination of massive imaging data. High-throughput screening (HTS) examines the effects of thousands of chemicals on cells and biological targets by combining picture collection with advanced image processing techniques. Image analysis is used to find potential medication candidates, evaluate their effectiveness, and tweak their properties.
2. Image analysis is essential for understanding cellular and molecular processes. It enables the extraction of quantitative data from pictures, such as cell shape, protein localization, and dynamic biological processes. This aids in characterizing therapeutic targets, identifying illness processes, and assessing the cellular effects of medications.
3. To extract detailed information from cellular pictures, High Content Screening (HCS) combines automated microscopy with cutting-edge image processing methods. Using image analysis tools, several cellular characteristics may be quantified at once, enabling researchers to analyse intricate cellular responses to medicinal therapies. The characterization of pharmacological mechanisms and the discovery of lead compounds have been sped up using HCS.
4. Image analysis has considerably enhanced pharmaceutical manufacturing's quality control procedures. Drug formulation flaws, contaminants, and variants can be found using automated inspection systems utilizing image analysis algorithms. As a result, there is a greater emphasis on automated inspections and high-quality pharmaceutical items are consistently produced.
5. In the pharmaceutical business, image analysis techniques have revolutionized medical imaging and diagnostics. The automated analysis and interpretation of medical pictures, including those from X-rays, CT scans, MRI scans, and molecular imaging data, is made possible by advanced algorithms. Accurate diagnosis and treatment planning are made possible by image analysis, which helps with illness identification, characterization, and quantification.
6. Through computational pathology and digital pathology, image analysis has changed how pathology practices are carried out. Pathologists may take advantage of automated disease marker identification, quantification, and classification by digitizing pathology slides and using image analysis algorithms. Decisions about diagnosis, prognosis, and therapy may be made more precisely and objectively thanks to image analysis.
7. Image analysis helps with personalized medicine by making it possible to extract quantitative data from photos of medical conditions. Image analysis assists in customizing therapies to particular patient features and optimizing therapy outcomes by analysing imaging data from individual patients, such as genetic imaging, and functional imaging.
In general, image analysis has changed the pharmaceutical sector by giving experts sophisticated tools for deciphering and analysing intricate imaging data. Drug development has been sped up, manufacturing quality control has been improved, medical imaging and diagnostics have been improved, and personalized medicine methods have been made easier. Pharmaceutical research, development, and patient care continue to benefit greatly from image analysis. Tech companies like ImageProVision have developed several software-based image processing and analysing solutions for pharmaceutical clients.
Read Also - Image Filtering Techniques in Image Processing
How Has Image Analysis Advanced Scientific Research?
Scientific research in a variety of fields has benefited greatly from image analysis.
Here are some examples of how image analysis has aided scientific research:
- Cellular and molecular biology:
Image analysis tools make it possible to examine tiny cellular and molecular processes. Researchers may examine cellular behaviours, spot anomalies, and comprehend biological systems by extracting quantitative data from pictures, such as cell counts, shape, and protein localisation.
- Medicine and Biomedical Research:
Image analysis is widely utilised in medical imaging, such as X-rays, CT scans, MRIs, and histopathology slides, in both medicine and biomedical research. It aids in the diagnosis of illnesses, the observation of treatment outcomes, and the tracking of the development of ailments. Algorithms for image analysis may evaluate tissue properties, identify abnormalities, segment organs or tumours, and support personalised therapy.
- Neuroscience:
Researchers can examine brain imaging data, such as fMRI (functional magnetic resonance imaging) and EEG (electroencephalography), to find patterns, areas of interest, connections, and activity changes linked to various cognitive processes and neurological illnesses.
- Ecology and environmental sciences:
By automating species identification and categorization, image analysis makes it possible to study ecosystems and biodiversity. It supports ecological modelling, habitat evaluation, and population monitoring. To track changes in the flora, land use, and marine life, researchers can gather useful data via remote sensing photos, satellite imaging, and underwater surveys.
- Astronomy and astrophysics:
The processing and interpretation of astronomical pictures obtained by observatories and space missions heavily rely on image analysis. Identification, measurement, analysis of star formation processes, and universe mapping are all aided by it. Algorithms for image analysis also aid in the discovery and characterization of exoplanets.
- Materials science:
Image analysis methods are used to examine the microstructure and characteristics of materials. Images of the materials may be examined by scientists using methods like transmission electron microscopy (TEM) and scanning electron microscopy (SEM). This aids in characterising flaws, comprehending material behaviour, and creating sophisticated materials with certain features.
- Social Sciences:
Image analysis has been used in anthropology, archaeology, and sociology, among other social science fields. Images may be analysed by researchers to investigate cultural artefacts, identify trends in human behaviour, and assess visual interpretations in historical contexts.
These are only a handful of instances illustrating how image analysis has aided scientific investigation into a variety of subjects, improving data extraction, interpretation, and comprehension in ways that were not before conceivable.
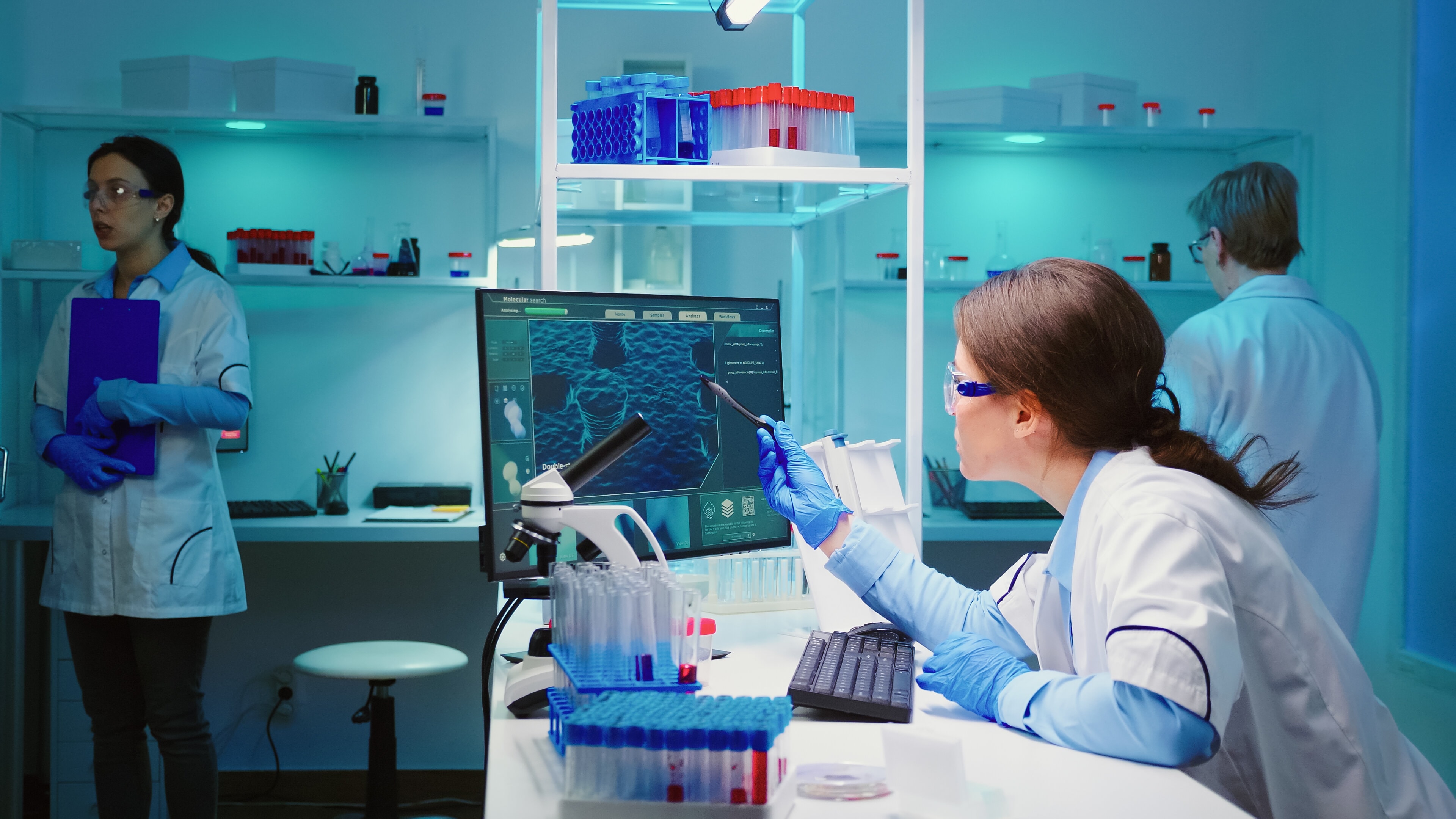
How Image Analysis Has Made Social Media Platforms More User-Friendly
The ability to identify graphics inside social media has been made easier by image recognition, which is performing well in this space. To find the proper photographs at unmatched speed and scale, image recognition technologies may scan social media sites for images and compare them to vast libraries. As a result, it provides businesses with significant advantages in terms of customer service. To provide an accurate description of the photo's content and to identify who is in it without being tagged, Facebook combined face recognition technology with automated text technologies in 2016. This function was implemented for the benefit of visually impaired persons.
Different Image Analysis Software Used:
For image analysis, there are several software tools and libraries that may be used to meet different needs and areas.
Here are a few examples that are frequently used:
- OpenCV:
OpenCV (Open-Source Computer Vision Library) is a well-known open-source computer vision library. It offers a large array of techniques and methods for analysing images and videos, such as feature extraction, object identification, and image processing. Numerous programming languages, including C++, Python, and Java, are supported by OpenCV.
- MATLAB:
High-level programming language and environment MATLAB was created especially for numerical computing and data analysis. For processing and analysing images, it offers a wide range of tools and functionalities.
- scikit-image:
Using NumPy, SciPy, and matplotlib as its foundation, scikit-image is a Python package for image processing. Filtering, segmentation, feature extraction, and other image analysis techniques are among the many methods and functionalities it offers.
- TensorFlow:
TensorFlow is a Google-developed, open-source deep learning framework. It provides lower-level capabilities and a high-level API (Keras) for creating and training neural networks for image analysis tasks including segmentation, object identification, and classification.
- PyTorch:
PyTorch is a well-known, open-source deep learning framework that is frequently used for image analysis. It offers a dynamic computational graph and enables operations like picture categorization, object identification, and segmentation.
- Cytoscape:
A framework for visualizing and analysing complex networks, including biological networks, is called Cytoscape. For network-based image analysis, it offers a variety of image analysis functions.
- CellProfiler:
Developed for high-throughput cell image analysis, CellProfiler is an open-source programme. It offers an easy-to-use user interface and a variety of image analysis modules to assess different cellular properties.
- ENVI:
ENVI (Environment for Visualising Images) is a commercial programme that is frequently used for the interpretation of remote sensing images. In order to complete tasks like picture categorization, change detection, and image interpretation, it provides extensive image processing and analysis capabilities.
- DeepLabCut:
DeepLabCut is a well-known open-source software toolkit created especially for animal behavioural analysis and markerless posture estimation. For the purpose of analysing and tracking animal movement in films, deep learning algorithms are used.
- ipvPClass:
The ipvPClass is an advanced version of the Particle Size Analyzer, rendering the analyses with speed, convenience, and accuracy. ipvPClass is a Microscopic Image Analysis system that includes a high-resolution camera & dedicated software which can be integrated with any microscope for the identification of particulate solids based on particle size, shapes, and textures within the microstructure of the sample.
These are but a few illustrations of software applications used in image analysis. The programme used will rely on the particular needs, the resources at hand, and the user's level of experience.
Read Also - Particle Size Analysis: Importance & Applications
Future of Image Analysis:
1. Deep learning has already made a big difference in image analysis, and it is predicted to keep getting better. Future developments might include more effective and potent deep learning architectures, enhanced training methods, and improved handling of sparse or noisy data.
2. As AI systems proliferate, there will undoubtedly be a rise in the need for explainable and comprehensible image analysis models. The development of techniques that offer transparency and insights into the decision-making process of AI models may be the subject of research, allowing users to comprehend and trust the findings. Many leading global tech companies like ImageProVision are operating in the physical testing space with an emphasis on image analytics.
3. There is a rising need for effective and low-latency image analysis algorithms due to the growing need for real-time picture analysis in applications like autonomous cars, robots, and surveillance systems. For real-time image processing on devices with limited resources, future developments may entail creating lightweight models and edge computing strategies.
4. Privacy protection and image security will be essential as image analysis becomes more common. Future studies may concentrate on creating privacy-preserving methods, such as differential privacy or secure federated learning, to protect personal information while facilitating efficient picture analysis.
5. Increased emphasis on fairness, prejudice reduction, and ethical issues will probably be seen in image analysis in the future. Researchers and practitioners will keep looking towards ways to deal with biases in training data and guarantee fairness in algorithmic results.
These are possible directions that could influence the development of image analysis. The real future of the area will be greatly influenced by developments in technology, data accessibility, and societal requirements.